Katherine Sytwu, Edward Barnard, David Prendergast
Recent advances in data-driven methodologies such as data mining, machine learning, and statistical modeling have enabled researchers to incorporate observations and technical intuition that may be difficult to analytically define into the experimentation process and extract out statistically-relevant behavior. Simultaneously, high-throughput synthesis and characterization have led to a deluge of scientific data that presents new challenges in both understanding information content and responding appropriately to focus on interesting regions or phenomena. This symposium will highlight the diverse opportunities and challenges in which data science can inform, augment, and improve experimentation and analysis for applications spanning autonomous materials discovery and synthesis, correlative and multimodal measurements, novel data analysis algorithms, and data infrastructure challenges that underpin implementation into experimental workflows.
Symposium Sponsor:
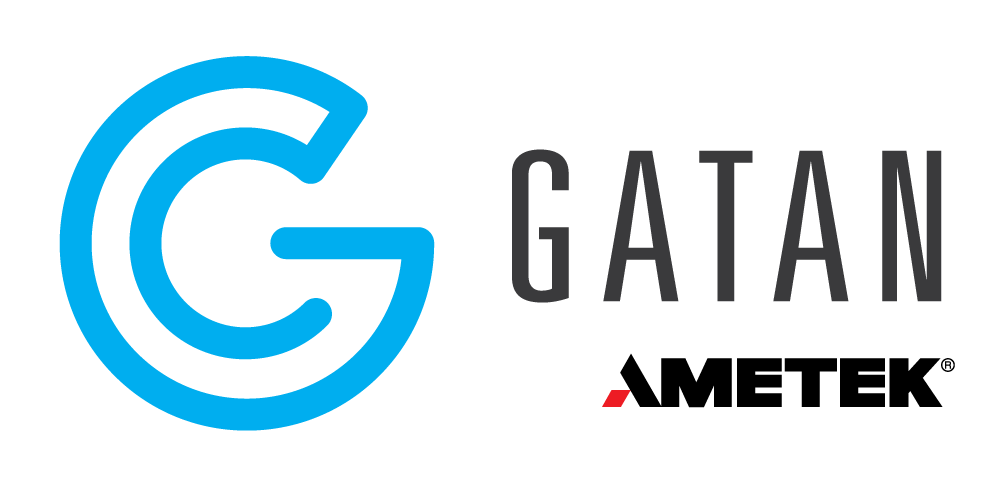
Symposium Location: B50 Auditorium
Symposium Schedule:
12:45 – 1:15 pm
Full-stack materials discovery and its discontents
Joseph Montoya, Toyota Research Institute
1:15 – 1:30 pm
Learning Design Principles for Perovskite Intergrowth Heterostructures Using High-Throughput Synthesis
Caravaggio Caniglia, Stanford University
1:30- 1:45 pm
Automatized recognition of Transition Metal Dichalcogenide (TMD) two-dimensional materials
Myriam Diatta, Berkeley Lab
1:45 – 2:15 pm
Incorporating spatial and temporal constraints into neural networks
Aditi Krishnapriyan, University of California, Berkeley
2:15 – 2:45 pm
Break
2:45 – 3:15 pm
Real Time Machine Learning in Microscopy and Spectroscopy
Mitra Taheri, Johns Hopkins University
3:15 – 3:30 pm
Machine Learning Prediction of Bond Valence from EELS Spectra of Cu Materials
Samuel Gleason, University of California, Berkeley
3:30- 3:45 pm
An artificial intelligence’s interpretation of complex high-resolution in situ transmission electron microscopy data
Xingzhi Wang, University of California, Berkeley
3:45 – 4:00 pm
Automating 4D-STEM Data Acquisition
Alexander Pattison, Berkeley Lab
4:00 – 4:15 pm
Seeing Through the Speckle: Multimodal Analysis of Medium-Range Order in Amorphous Rare Earth – Transition Metal Thin Films
Ellis Kennedy, University of California, Berkeley